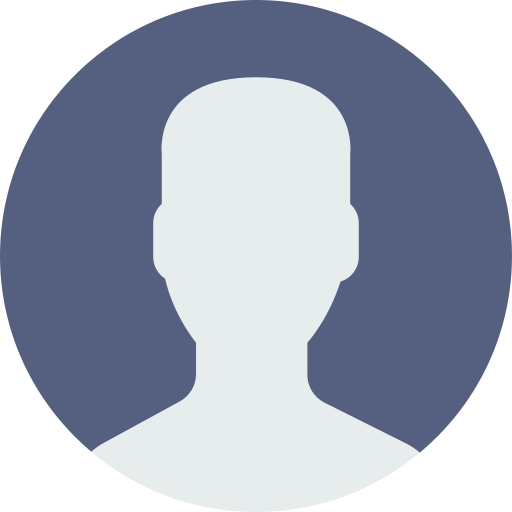
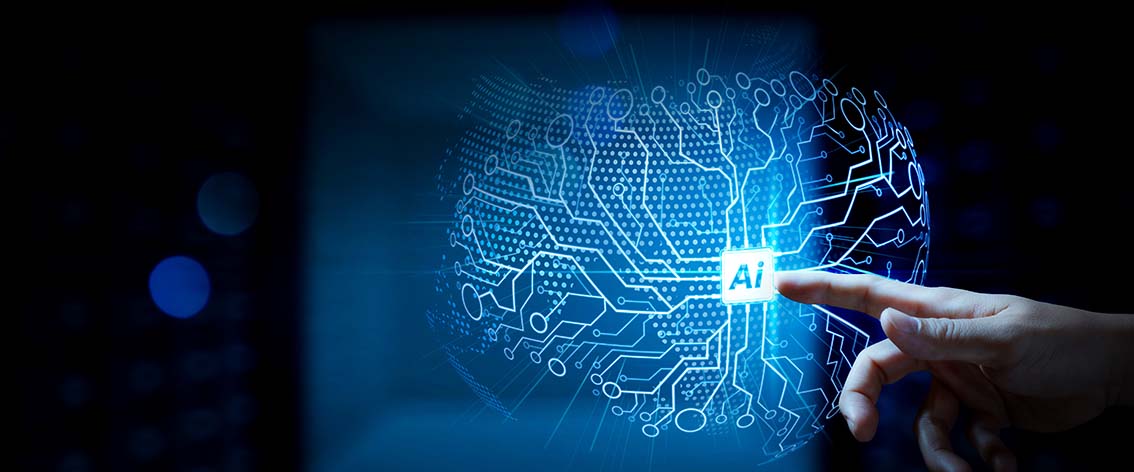
In 2006, the British mathematician Clive Humby coined the famous sentence, “Data is the new Oil”. But data alone is a jumble of numbers. Like an artist transforming a mass of clay into a beautiful sculpture, someone—or something—must carve meaning out of these figures. Enter artificial intelligence, or AI, which has been instrumental in making sense of the ever-growing amount of data that we collect and store every day.
In particular, the development of a machine learning (ML) technique called deep learning has revolutionized all sorts of domains from games like Chess and Go to the very human skills of natural language processing, speech and image recognition. It’s no wonder that the airline industry, like many others, has turned to Machine Learning to sift through its mounds of data to help model customer choices, travel demand, or willingness to pay.
Bringing human knowledge and machine learning together
But deep learning alone, like all technologies, has its limitations. For one thing, the conclusions reached by artificial intelligence are only as good as the data it uses, and accessing vast amounts of accurate and curated data is often difficult, expensive, or impractical. Secondly, the neural networks that make deep learning possible require vast amounts of hardware and energy to train. And finally, there is an inherent weakness in deep learning models: since AI systems train themselves over time, the decisions they reach can be opaque. This makes deep learning models unsuitable for heavily regulated industries, where transparency and accountability of the decision-making process are of utmost importance.
To address these limitations, AI researchers are now focusing on the development of Knowledge-Aware AI systems (KAAI). By integrating human knowledge with machine learning, we can significantly reduce the amount of data required, increase reliability, and build more transparent machine learning systems.
For example; software for a finance company will look drastically different than software for a pharmaceutical company or for an airline company. By integrating “domain knowledge” or knowledge from human subject matter experts, with machine learning models, we can design more refined deep learning models.
Knowledge-Aware Artificial Intelligence
How can Knowledge-Aware AI benefit airlines?
At Amadeus, we have a strong track record in building domain knowledge models for the airline industry, especially when it comes to modeling customer choice, predicting travel demand or traveler’s willingness to pay for different offers. Most recently, we developed a recognized expertise in modern AI technologies including deep learning, recommender systems, reinforcement learning or generative adversarial networks. That’s why we are in a very good position to exploring hybrid machine learning, which aims to combine the efficiency of human domain knowledge models with the flexibility of the pure data-driven machine learning approaches. With this hybrid approach, we can get the best of both worlds.
For example, we recently published a study showing how human airline domain knowledge can be used to generate the tremendous amount of data required for training an offline deep reinforcement learning model to improve price optimization in airline revenue management systems. To go further, we’re currently working on a hybrid model for price optimization in Amadeus Airlines Revenue Management, combining domain knowledge and machine learning.
This is just the beginning, thanks to the richness of data and our airline expertise, we can reach the moon. You will soon hear about our move towards Knowledge-Aware Artificial Intelligence in airline revenue management. Stay tuned!
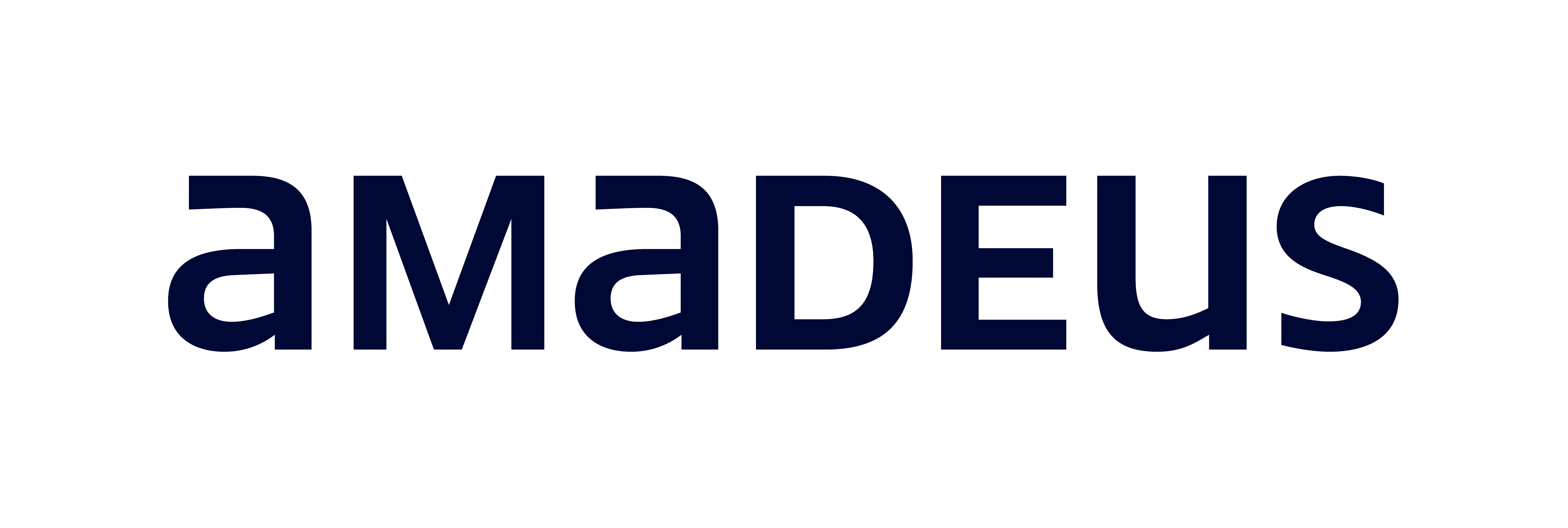